-
Complex information networks are ubiquitous with increasingly voluminous and diverse data: examples include social networks, medical health records, stock market, disaster response, and environmental science. Complex, partial, unstructured and time-varying multimodal data pose significant new challenges to knowledge discovery. Healthcare is an excellent example of a data offering all these challenges: patient health records, consisting of imaging data (CT scans, MRI, pathology images), physiological measurements, physician notes, disease states, treatment history including medication, and outcomes (well-being of the patient). The spatial variations (e.g., in 2D/3D images) and temporal behavior (response to medication, changes in imaging and other measurements) are further complicated because data sampling is not uniform in any of these dimensions. The primary mission of the Center for Multimodal Big Data Science is to address the grand challenge of developing novel computational methods leveraging image analysis, natural language processing, machine learning, system identification and database technologies to discover significant knowledge to provide a transformative impact on a broad spectrum of applications.
A central component in this research effort is the BisQue image management and analysis platform that has been developed in the lab over the past 10 years. BisQue specifically supports large scale, multi-dimensional multimodal-images and image analysis. Metadata is stored as arbitrarily nested and linked tag/value pairs, allowing for domain-specific data organization. Image analysis modules can be added to perform complex analysis tasks on compute clusters. Analysis results are stored within the database for further querying and processing. The data and analysis provenance is maintained for reproducibility of results. BisQue can be easily deployed in cloud computing environments or on computer clusters for scalability. The user interacts with BisQue via any modern web browser.
The interdisciplinary research projects built on top of BisQue span four main areas: marine science, plant biology, materials science, and healthcare.
LIMPID/BisQue + IDEAS Joint Workshop, Feb 3-5, 2020
The center we will be hosting the second LIMPID/BisQue workshop on Scalable Image Informatics. This is a joint meeting with the workshop on Applications of Machine Learning and Scalable Image Informatics to Materials Discovery, held as part of the NSF-sponsored IDEAS program on Data-Driven Approaches to Materials Discovery, at the University of California at Santa Barbara. Participation is by invitation only and we are expecting over 80 registered participants.
The three day joint workshop will take place at UCSB from February 3-5, 2020. The workshop will start at 8:00 AM on Monday, February 3rd and adjourn by 5:00 PM on Wednesday, February 5th.
This workshop will bring together researchers from Academia and National Laboratories who are developing or have adopted new experimental methods and measurement tools that provide very large and rich data sets, with early adopters of machine learning as well as leaders in the machine learning community. The main objective of the LIMPID/BisQue workshop is to identify key requirements for scalability and sustainability of software infrastructures to support multimodal data analysis and machine learning in diverse application areas such as life sciences, marine science, materials and health sciences. A key objective of the IDEAS workshop is to connect researchers in the data sciences field with domain researchers to foster a dialogue and establish the state-of-the-art in the application of machine learning to materials discovery related disciplines, and identify (i) the potential impact of, and opportunities for, data sciences in materials, with an emphasis on integrating experimental and computational materials; and (ii) the grand challenges at the intersection of materials discovery and machine learning.
Monday will focus on sustainable software infrastructure for multimodal informatics and machine learning for image applications. Tuesday will address data-driven approaches for advanced materials applications, effectively educating a workforce of data-driven innovators, and high-throughput approaches in computational mechanics. Wednesday will focus on accelerating data-intensive research with a range of applications, and AI and ML for high-dimensional and multi-scale datasets.
Organizers
Samantha Daly, Mechanical Engineering, UCSB; B.S. Manjunath, Electrical and Computer Engineering, UCSB; Tresa Pollock, Materials, UCSB
-
-
Bio-Image Informatics
EM2 data and Cellular segmentation
Material Science & Structural Analysis
Dream3D and Structural Analysis
Healthcare
Patient monitoring and analytics
Marine Sciences
Underwater image segmentation and classification
Recent Papers
(None)
- “Structure-Forming Corals and Sponges and Their Use as Fish Habitat in Bering Sea Submarine Canyons”,PLoS ONE, vol. 7, no. 3, Mar. 2013.
-
Frontiers in plant science, vol. 2, Jul. 2013.
-
Springer, pp. 56--66, Copenhagen, Denmark, Nov. 2015.
-
IEEE Proceedings, pp. 1--9, Nov. 2016.
-
Springer, ECCV-ACVR, pp. 178--194, Amsterdam, The Netherlands, Nov. 2016.
-
2017.
-
Feb. 2016.
-
2017.
-
Oct. 2016.
-
Dec. 2016.
-
2016.
-
Jun. 2017.
-
Sep. 2017.
-
2018.
-
Sep. 2018.
-
Sep. 2018.
-
Jan. 2019.
-
Apr. 2019.
-
Jun. 2019.
-
Oct. 2019.
-
Oct. 2019.
-
Jan. 2020.
-
2021.
-
Apr. 2021.
-
Sep. 2021.
-
Apr. 2018.
-
Nov. 2018.
-
Aug. 2019.
-
Sep. 2015.
-
Dec. 2014.
-
Jun. 2015.
-
Proceedings of Machine Learning Research, Nov. 2020.
-
Jun. 2018.
-
May. 2018.
-
Feb. 2019.
-
Nov. 2019.
- “Learning Weighted Submanifolds with Variational Autoencoders and Riemannian Variational Autoencoders”,Nov. 2019.
-
Nov. 2021.
-
Aug. 2020.
-
Apr. 2020.
-
May. 2020.
- “VSGNet: Spatial Attention Network for Detecting Human Object Interactions Using Graph Convolutions.”,Mar. 2020.
-
Dec. 2018.
-
Mar. 2019.
-
Aug. 2020.
-
Jun. 2019.
-
Mar. 2019.
-
Sep. 2021.
-
Nov. 2018.
- “3D Grain Shape Generation in Polycrystals Using Generative Adversarial Networks”,2021.
- “EBSD-SR: Super-Resolution for Electron Backscatter Diffraction Maps using Physics-Guided Neural Networks”,2021.
-
2021.
-
Apr. 2020.
-
Aug. 2021.
-
nt J Comput Vis, vol. 131, pp. 1367–1388, Jul. 2023.
Research
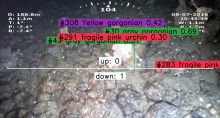
BisQue has been used to manage and analyze 10 hours of unexplored ocean habitats using underwater remotely operated vehicles and 23.3 hours (884GB) of high definition video from dives in Bering Sea submarine canyons to evaluate the density of fishes, structure-forming corals and sponges and to document and describe fishing damage.
We propose a deep learning model to identify the characteristic differences in Computation Tomography (CT) scans between COVID-19 and other similar types of viral pneumonia
We propose a novel and efficient algorithm to model high-level topological structures of neuronal fibers.
We propose a novel weakly supervised method to improve the boundary of the 3D segmented nuclei utilizing an over-segmented image.
The Deep Eye-CU (DECU) project integrates temporal motion information with the multimodal multiview network to monitor patient sleep poses. It uses deep features, which slightly improved the patient sleep pose classification accuracy (when compared to the performance of engineered features such as Hu-moments and Histogram of Oriented Gradients (HOG)). The DECU also uses principles from Hidden Markov Models (HMMs) a popular technique in speech process. It leverages pose time-series data and assumes that patient motion can be modeled using a “state-machine” approach. However, HHMs are limited in their ability to model state duration. In a high level analysis, state duration is used to distinguish between poses and pseudo poses, which are transitory poses seen when patients move from one position to another. The DECU framework (system and algorithms) are currently deployed in a real medical ICU at Santa Barbara Cottage Hospital where study volunteers have consented to the study.
The Eye-CU project incorporates a multiview aspect of the network to successfully remove the complex and prohibitively expensive pressure mat. This work uses purely visual rgb and depth sensors position at relatively different locations (i.e., multiview). Eye-CU learns the weights the contribution of each sensor and view via couple-constrained Least-Squares (cc-LS) modality trust estimation algorithm. The Eye-CU system in combination with cc-LS successfully match the performance of the MEYE network while and reliably classifies patient poses in challenging scene conditions (variable illumination and various sensor occlusions).
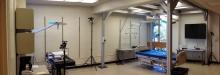
For instance, the MEYE (multimodal ICU ) network focuses on the detection of patient sleep poses using multimodal sensor network data: cameras (rgb, depth, thermal), a pressure mat (flexible sensor array), and room environmental sensors (temperature, humidity, sound). The multimodal data allows the algorithms to deal with challenging scene conditions (partial sensor occlusions and illumination changes). The rooms sensors are used to trigger and tune modality weights.

The Multimodal Multiview Network for Healthcare is a collaborative effort between researchers from the Electrical and Computer Engineering Department at the University of California Santa Barbara and the intesivists and medical practitioners from the Medical Intensive Care Unit (MICU) at Santa Barbara Cottage Hospital. The Objective of the research is to improve quality of care by monitoring patients and workflows in real ICU rooms. The network is non-disruptive and non-intrusive and the methods and protocols to protect and maintain the privacy of patients and staff.
In this project, we integrate the Dream.3D software package into BisQue. Dream.3D is an open and modular software package that allows users to reconstruct, instantiate, quantify, mesh, handle and visualize microstructure digitally. By integrating it with BisQue, Dream.3D runs can be managed from any computer with a web browser, due to BisQue's web-based nature. Similarly, results and visualizations can be easily shared with collaborators right from a web page, without any software installation. In addition, analysis can be scaled out to run on compute clusters for faster exploration of parameter spaces. Furthermore, BisQue adds provenance tracking, allowing the Dream.3D user to understand exactly the data flow of inputs and outputs and the analysis settings. This greatly improves reproducibility and the understanding of analysis history.
The BisQue image analysis platform was used to develop algorithms to assay phenotypes such as directional root-tip growth or comparisons of seed size differences.
Sponsors
News
-
2022-02.
We are co-organizing a computational challenge at the ICLR 2022 workshop of geometry and topology. The goal is to push forward the field of computational differential geometry by asking participants to contribute statistics and learning algorithms on manifol
-
2021-11.
BisQue 2 has recently launched with a beta release! This new version of BisQue has a new storage backend and fully runs on Kubernetes. Active BisQue users should begin migrating any data stored on BisQue to BisQue 2, as BisQue will eventually be deprecated.
-
2021-11.
Despite vaccines being readily available for almost every age group, Covid-19 still remains a fatal disease if not detected early enough. As such, two Covid-19 detection modules are making their way to BisQue's cloud platform in the near future.
-
2021-11.
Normal Pressure Hydrocephalus (NPH) is a disorder in which ventricles in the brain swell from an accumulation of excess cerebrospinal fluid. This disorder can lead to difficulty in walking, thinking, and other daily functions.
-
2021-11.
The BioShape lab has been awarded an NIH R01 grant for biological shape reconstruction. The lab aims to introduce geometric and deep learning methods to enhance 3D biological shape reconstruction.
Collaborators:
-
2021-11.
BisQue has now moved its entire orchestration system to align with the latest software trends for distributed systems. With the move to Kubernetes, BisQue is now highly available (fault tolerant) and scalable across multiple nodes.
-
2020-02.
An accurate 3D cell segmentation module utilizing a 3D U-Net based neural network, a 3D watershed algorithm applied to a probability map, and CRF refinement has been integrated into the BisQue cloud platform.
-
2017-12.
UCSB researchers given the award from NSF’s Office of Advanced Cyberinfrastructure to build a large-scale distributed image-processing infrastructure (LIMPID) through a broad, interdisciplinary collaboration.
-
2020-02.
The center we will be hosting the second LIMPID/BisQue workshop on Scalable Image Informatics.
Software and Resources
LIMPID/BisQue
Bisque is an advanced image database and analysis system for multimocal images. It supports large scale image databases, flexible experimental data management, metatdata and content based search, analysis integration and knowledge discovery. The system is based on scalable web services.
Read more about LIMPID/BisQue in this Outreach Article.
Resources
Github Code : https://github.com/UCSB-VRL/bisqueUCSB
Github Pages: https://ucsb-vrl.github.io/bisqueUCSB
Contact
B.S. Manjunath
Distinguished Professor, Electrical and Computer Engineering Department
Director, Center for Multimodal Big Data Science and Healthcare
University of California
Santa Barbara, CA 93106-9560
Tel: (805) 893 7112
E-mail: manj [at] ucsb [dot] ed